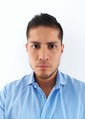
Isaac Monroy
Universidad Anahuac Queretaro, Mexico
Title: Paracetamol degradation model in water by the photo-Fenton process with artificial neural networks
Biography
Biography: Isaac Monroy
Abstract
Data-based models are an alternative to first-principle kinetic models to simulate chemical and biochemical processes that have been barely exploited in the modelling field. These models are constructed with the aid of Machine learning methods such as Artificial Neural Networks (ANN), which have been applied in different research areas. In this work, ANN have been used for modelling the Photo-Fenton process and monitoring both the paracetamol (PCT) photodegradation and the organic matter mineralization in water. PCT has been identified as an emerging and recalcitrant contaminant in wastewaters, found in European Sewage Treatment plants (Ternes, 1998) and reported as detrimental to animal and human health. In order to degrade it, Advanced Oxidation Processes (AOPs), among them Photo-Fenton, have been successfully applied. However, process models have not been produced aimed at practical monitoring and soft-sensing of contaminant concentrations from unexpensive on-line data. Thus, experimental data were collected from a 15L pilot plant, where assays under different initial conditions (Fe(II) and H2O2 concentrations) were performed. Eight on-line measured process variables plus Fe(II) and H2O2 initial concentrations were the input to the ANN. Model outputs were compared to the experimental off-line measured variables such as Total organic carbon (TOC), H2O2 and PCT concentrations, obtaining good but not satisfactory correlation coefficients between both of them. Nevertheless, the application of PCA statistical method to the original data and previous to ANN improved the correlation results to 0.95 in average, which evidenced the construction of a reliable data-based model for the PCT degradation in water by Photo-Fenton.